Understanding Donor Characteristics to Improve Allogeneic Cell Therapy Outcomes

By Erin Harris, Editor-In-Chief, Cell & Gene
Follow Me On Twitter @ErinHarris_1
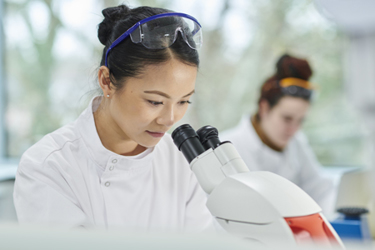
Equity within the cell and gene therapy space and reported characteristics such as race and ethnicity can impact data quality. As such, efficiencies in donor selection reduce uncertainties about human leukocyte antigen (HLA) and improve patient outcomes. I caught up with Abeer Madbouly, Principal Bioinformatics Scientist and Joy Aho, Director, Product Management Be The Match BioTherapies to learn more.
How can proven transplant infrastructure assist in delivering cell and gene therapies?
Joy Aho: The infrastructure to support transplant parallels the needs for cell and gene therapies in many ways, starting with the identification of therapy-matched donors. In support of transplant, we have had to not just grow a registry of volunteer donors, but also one that has the diversity to meet the needs of the patient population. Once a donor is identified and vetted for eligibility and suitability, an infrastructure to collect the cellular material with high quality and consistency is required. As transplant has grown, we have adjusted our collection network to align with the location of the donor population as well as to optimize product quality. Finally, managing the logistics to ensure the product arrives on time while maintaining temperature requirements is critical. We have built relationships with couriers, customs, and the emergency management community to ensure the product continues to move no matter what is going on in the world.
All of these elements are also critical to cell and gene therapies that leverage cellular starting material. The infrastructure built for transplant can help ensure the scalability of these therapies as more move towards later clinical phases and commercialization. This scalability will be critical to ensure all patients in need are able to receive their life-saving cell and gene therapy.
Explain the complexity of human leukocyte antigen (HLA) in cell selection (including reported race, ethnicity, and the impact on data quality
Abeer Madbouly: Donor/recipient HLA matching is crucial to the success of HCT. The HLA gene system is the most polymorphic in the human genome and has experienced selective pressures over generations of human evolution. This means that the different immune responses that help humans survive pathogens, migration patterns, wars, colonization movements, natural catastrophes, or changes in procreation patterns in different areas of the world have helped shape the HLA alleles and haplotypes in every region of the world, depending on the selective pressures at play. Additionally, some world populations have been exposed to several patterns of admixture resulting in the emergence of populations such as African-Americans with African and European admixture and Hispanic/Latino populations with multiple degrees of Indigenous-American, European and African admixtures. All these factors contribute to the formation of certain HLA patterns particular to each geographical area in the world. Self-reported race and ethnicity is one of the most effective ways to capture population-specific HLA ancestral information. Collecting this data from millions of volunteer donors that join the registry helps us build population-specific HLA reference data that is used for accurate donor/recipient match predictions, modeling match rate projections for multiple patient populations, and resolving ambiguities and gaps in HLA data which effectively improves our HLA data quality. These ambiguities exist because of the evolution of laboratory HLA genotyping methods as well as continuous clinical research showing added benefit in matching more HLA genes than decades ago. To be able to provide match predictions on multiple HLA loci, HapLogic, the BTM matching algorithm, is able to accurately and effectively fill gaps that exist in some of our donors data utilizing the HLA reference datasets that we built from our donors' HLA and reported race and ethnicity information.
Why is the use of machine learning important in informing cell/source selection?
Abeer Madbouly: Multiple machine learning (ML) algorithms have been built by the CIBMTR Bioinformatics and Statistical teams to improve several aspects of the cellular therapy experience, including optimizing cell/source selection by predicting potential donor pools that are available with particular profiles that lead to a successful transplant. One of the most effective measures to predict donors' availability is the donor readiness score (DRS), developed using a multi-dimensional ML algorithm that takes into account multiple donor features like age, gender, geographical location, race, ethnicity, and many more. This score has proven accurate and effective in producing a candidate donor pool for cell/source selection that would be available when called to donate. Other ML models have been developed to predict post-transplant survival under different HLA matching scenarios, transplant regimens and donor characteristics. The team continues to develop these models to improve multiple stages of the cellular therapy experience.