The 4 Ways AI Implementation Is Changing The Future Of Precision Medicine
By Apeksha Damania and Bill Woywod, Guidehouse
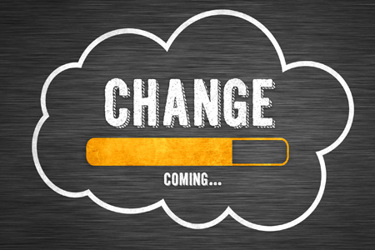
Part 1 of this two-part series examined how recent advances in for oncology diagnosis and treatment preview the full potential of precision medicine. This article, Part 2, shares insights on the challenges the industry must overcome before individualized healthcare can be fully realized.
The premise of AI-integrated precision medicine relies on a computational system having a large amount of health and wellness data points about a person, such as their genome, metabolic profile, microbiome composition, physical activity level, nutrition, sleep activity, etc., as well as the data and understanding of a particular disease pathway in that person. Synthesizing this data, AI-based precision medicine tools will soon create a tailored treatment regimen to optimize the individual’s response.
However, the life sciences industry must overcome significant challenges and answer some important questions before drug therapies can take full advantage of precision medicine to meet the unique needs of patients.
AI/ML algorithms depend on access to vast amounts of verified and validated data in order to learn and continuously improve. Consequently, scientists still need to determine how useful AI platforms – such as CURATE.AI, which was discussed in Part 1 – will be in cases where data is unavailable or difficult to validate as accurate. For example:
- How can AI/ML be applied to therapeutics for rare diseases or patient characteristics for which extensive data is not available?
- For therapies in general, how relevant and applicable will AI/ML-fueled technologies be across geographies, especially considering the vast disparities in terms of data collection and access, national wealth and resources, and patient access to healthcare?
- What infrastructure and regulatory requirements will be needed at an institutional and global level for AI models to be convincingly applied across multiple therapeutic areas?
To address these vital questions and bring precision medicine reliably and equitably to fruition, the industry will need to be prepared to shift priorities, adapt, and progress.
1. Real-World Evidence Is Coming To The Fore
Particularly when it comes to AI-driven medical technologies, regulators around the world already are increasingly calling for more post-market surveillance and the collection of real-world evidence (RWE). This is both rational and appropriate. In a precision medicine world, RWE will become even more important as it provides the information needed for an AI algorithm to identify and optimize individualized therapies.
While the overall importance of RWE grows, so too will the importance of clinical trials, but for a different reason. By definition, the RWE data used to train the AI/ML models for precision medicine will be built on historical data. Consequently, the newest therapies will lack representation in the pool of RWE data, so AI/ML algorithms will have insufficient data to recommend them. As a result, clinical trials will maintain their role in providing the initial evidence needed to spur acceptance and adoption of new therapies. However, this will not happen at the expense of RWE and AI – rather, AI-based approaches will depend on access to data in sufficient quantities to train a model, and such data is only available if the initial clinical trials are successful. Then, once a new drug is sufficiently adopted, it will appear in the RWE data sets on which precision medicine relies.
In other words, the relationship between traditional clinical trials and RWE-based precision medicine will be increasingly symbiotic, with the AI feeding off and improving the utility of information generated. This means pharmaceutical companies must have a comprehensive data and evidence strategy that encompasses traditional and AI-based approaches to extract maximum value from both and be better positioned to achieve marketplace success.
Meanwhile, to fully realize the promise of precision medicine, life sciences companies must develop more mature methods of standardizing data collection and availability and build a robust, interoperable infrastructure to extract and integrate information across systems and providers. As such, the data aggregation and data engineering that power AI will need to become a discipline and industry of its own. In many cases, RWE data could conceivably be linked across a patient population, but first a common understanding of what data should be captured and how to assess and verify its purity must be determined.
2. The Role Of The Data Scientist Is Evolving
Another important change will be to the practice of data science itself. Precision medicine requires a different type of data scientist. Historically, clinical trials have relied on inferential statistics, which is instrumental in determining whether a pattern observed in a study is generalizable to the broader population or not. While that skillset will continue to be necessary in precision medicine – for example, in guarding against spurious results due to a multiple comparisons error – it is no longer sufficient on its own. Data scientists that understand machine learning, and in many cases deep learning and other AI approaches, will be essential.
Rather than inferring whether a difference between two populations is likely to be real or not, the data science approach employed in precision medicine is more practical: Can we more accurately pair highly specific subgroups of patients with similarly niche therapeutics in a manner that optimizes patient outcomes? This is a classification task rather than an inference task, and the skillset required is correspondingly different. Data scientists must be adept at implementing machine learning, including learner selection, dimensionality reduction, model validation, and hyperparameter tuning, which, in many cases, is distinct from the skillset used in classical inferential statistics.
3. In Tandem, The Role Of The Physician Is Changing
Healthcare professionals are meeting the progress of AI in precision medicine with mixed emotions – at once excitement for all the benefits it promises and concern it will potentially sideline them, or even render them obsolete. With this in mind, it is important to note: The role of AI in precision medicine, especially as it pertains to the diagnostic process, is to be seamlessly embedded into clinical workflows to support clinicians and enable them to focus on what they do best. In as much as the AI/ML algorithms excel in terms of execution of tasks that rely on pattern recognition, the day-to-day reality of medicine depends on the synthesis and holistic assessment of large amounts of disparate, often incomplete information. This is where the human mind is more capable than an algorithm. This means the future success of AI precision medicine rests in the ability to synchronize it with proven medical knowledge and a deep understanding of clinical workflows.
4. Precision Medicine Diagnostics Will Become Its Own Discipline
Aside from the therapeutics, pharmaceutical companies should anticipate that precision medicine diagnostics will grow into its own discipline. Liquid biopsy, whole exome sequencing, next-generation sequencing, etc., will all be critical in providing the predictor variable data needed to implement individualized healthcare. For pharmaceutical companies, they represent important complementary technologies that should be taken into account in market development efforts.
For example, the appropriate prescribing of a niche rare disease therapeutic may be dependent on the results from a similarly advanced AI-based precision diagnostic. In such a case, it may make sense for the pharmaceutical company to merge with a diagnostics company to facilitate broad access to the diagnostic.
While these precision diagnostics represent compelling market development complements to highly targeted therapeutics, they also represent significant business opportunities in their own right.
Fast-Forwarding To The Future
These are just a few of the overarching changes the life sciences industry will likely undergo as AI-fueled innovations continue to transform medical technology and redefine therapeutic possibilities. Those pharmaceutical companies that anticipate the shift and prepare for the promise of precision medicine to come to fruition will be best set to join the next generation.
About the Authors:
Apeksha Damania, Ph.D., is a consultant within the Life Science practice at Guidehouse. She has more than seven years’ research experience in translational medicine, with technical expertise in regenerative medicine, biomaterials, tissue engineering, and drug delivery approaches. Her areas of functional expertise include market access, evidence-based research and analysis, competitive assessment, and publication and communication strategy.
Bill Woywod is an associate director in health at Guidehouse. He has over 10 years’ experience leading advanced analytics and data science initiatives across the healthcare industry, including biopharma, medtech, and public and commercial payer. His areas of technical expertise include supervised and unsupervised machine learning, claims data analytics, rate setting, natural language processing, and econometrics. Woywod founded and leads Guidehouse's COBRA expert community, a collective of experts at the intersection of life sciences and data science focused on thought leadership and continuous innovation.