AI Vs. Humans In Novel Drug Target Identification
A conversation with Mihee M. Kim, Ph.D.
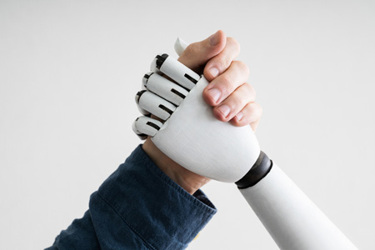
Facing an increasingly diminished environment for basic research and novel drug target identification, pharmaceutical developers are favoring to invest in technology, including artificial intelligence, over people.
The trend causes concern for Mihee Kim, Ph.D., a biologist and consultant who has led discovery teams at ReCode and CSL Behring, not because she sees it as a threat, but because things appear to be happening out of order, and it puts the whole endeavor at risk. Deploying half-baked technology could erode trust in artificial intelligence when it fails. That could set back new discoveries or even prevent them from happening altogether.
It's common to hear industry leaders describe technology as supplemental tools meant to enhance productivity and discovery, not replace humans who have historically done that work. But from Kim’s vantage point, that’s exactly what is happening.
We asked her to help us frame artificial intelligence’s most valuable role in drug target discovery right now, and imagine how the mix between humans and machines should look as the technology evolves.
Where do you think AI adds the most value in the drug discovery process? Where does it fall short?
As machine learning algorithms get ever more sophisticated, it is hard to pinpoint where the biggest breakthroughs will come from. In the forefront is the predictive power of AlphaFold, and it could be any day now we get our first small molecule that was solely discovered via an in-silico screen.
Additionally, in the near term, it will transform our ‘omics landscape, helping make connections and distilling large data into better models. This means the target identification process will likely be improved. The addition of machine learning in the world of ‘omics is part of an evolution in which scientists had set the stage many years ago.
Where value is added today is not in the predictive power but in the generation of a diversity of ideas and hypotheses that can be vetted by a scientist who has the ability to test them empirically. The time between new hypothesis and testing can be significantly shortened and be improved with each interaction.
To date, most novel discoveries or disruptive therapeutic platforms have been edge cases. For example, the discovery of CRISPR/Cas9 came through the observations of bacterial anti-viral defenses. It is not clear whether we can get to a point where AI can be trained to be predictive on these edges.
To add to the complication, biological experiments can be difficult to replicate. Mammalian cell lines can have genetic drift, mice may behave differently because of the differences in environmental factors from Maine to Colorado, etc. Often, these subtleties are not captured, or we color the data with our biases. Valuable data may be considered negative and dismissed as an error. For us to truly get to the next level, where machine learning would be valuable, we have to agree as a collective to be more agnostic on how we tag and categorize data, to follow models like those set by ‘omics scientists. A lot of it will depend on the databases and the curation of the data that these training sets or pre-training sets are fed.
What gets lost when companies downsize or sideline basic research teams in favor of AI-generated pipelines?
Trust in the systems gets lost if we abandon basic research teams in favor of an entirely-AI system.
Over the years, I have seen the hype cycle damage the reputation of amazing technologies. Eventually, they do always win out, but these wild swings cause huge inefficiencies in the industry. Wouldn’t it be great if just this one time, we could embrace a technology like AI with our eyes open so we can start working on the fundamentals to make it succeed?
Drug hunters are creative, risk-taking individuals. Will AI be able to preserve that level of creativity? Or will it be best suited for generating pipelines based on already successful drugs? A common lament among so many early research scientists is that they had an idea or project that was cut for business reasons. Will AI-generated pipelines be skewed for profitability? Will we be inundated with marginally better me-too’s to increase the probability of clinical success?
Are we at risk of AI models, trained on legacy data sets filled with expired assumptions and blind spots, reinforcing old biases in target discovery?
Yes and no. It truly depends on how vigilant we are in preventing these biases — basically, deploying humans to make sure we are avoiding them. Data that has been well curated and easily defined will likely be more evergreen than others. For example, RNAseq has a standard nomenclature and output. However, to continue the theme of this conversation, we would need more data to discover new things. We need data regarding people in different disease states, more data on people of different genetic backgrounds, and more data on people of different ages.
Some of our legacy data sets are based on qualitative data or unseen data of “stuff that was tried but did not work.” It is difficult to imagine how we would train a system on these assumptions and then somehow avoid those assumptions.
How do you react when you hear people say that AI is helping to democratize discovery by processing data faster than any human team ever could?
I am puzzled when I hear this, because what part is being democratized? I agree with the idea that drug discovery is an inefficient process, and that AI has the ability increase the efficiency. However, will everyone have access to everyone’s data? Will there be a new consensus on how we share experimental data, to improve these systems? How are we managing AI resources and is access to those free? Is democratization tied to cost or access or both?
What does an ideal hybrid model look like?
Scientists who understand how to use AI effectively will start replacing those scientists who do not. It will be an essential tool, just not the only tool. There will always be a need to generate more data if for no other reason than to better refine and improve the machine learning algorithms. Ultimately, a scientist is still accountable for the experiments, as a human is needed for the reality check. It is an exciting time when human intelligence can be enhanced by the artificial.
About The Expert:
Mihee Kim, Ph.D., is a scientist and independent consultant and formerly the executive director of discovery biology at ReCode Therapeutics. Previously, she was the head of gene therapy research at CSL Behring and, before CSL, worked for Janssen, now Johnson & Johnson. She received her Ph.D. in biology from Harvard University and completed undergraduate studies at Columbia University.