AI Integration In Drug Manufacturing: GMP Insights For Operational Excellence, Regulatory Compliance
By Josefine Sommer, Christopher Fanelli, Eva von Mühlenen, Michelle Gandolfo, and Julea Lipiz, Sidley Austin LLP
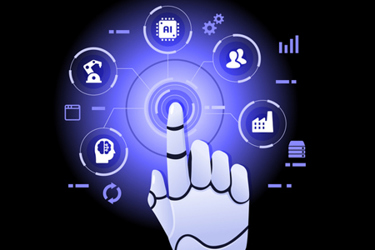
In the pharmaceutical industry, the integration of artificial intelligence (AI) and machine learning (ML) into drug manufacturing processes is redefining how drugs are manufactured and controlled for quality. U.S. FDA Commissioner Califf recently stated that “AI has the potential to enable major advances in the development of more effective, less risky medical products.”1 AI and ML in good manufacturing practice (GMP) settings offer unprecedented opportunities to enhance operational precision, efficiency, accuracy, and compliance through advanced analytics and automation in a highly regulated field. However, they also introduce complex regulatory challenges that need to be navigated carefully.
This article provides insights into a variety of use cases on the application of AI and ML in GMP settings and useful considerations for manufacturers using AI in quality to demonstrate GMP compliance.
Innovative Use Cases: Demonstrating AI Integration Into GMP Processes
AI/ML technologies excel at analyzing vast data sets more quickly and accurately than human capabilities allow, identifying patterns and predicting outcomes that are critical to maintaining the rigorous quality standards required in drug manufacturing. Many use cases, from streamlining processes across drug discovery to production, including predictive maintenance, personalized medicine, and real-time quality control,2 demonstrate the transformative potential of AI and ML in drug manufacturing. However, they also underscore the importance of developing robust regulatory frameworks to ensure these technologies are implemented safely and effectively.
- In the realm of aseptic processing, AI systems scrutinize every step of the manual vial filling process, analyzing each segment to detect potential contamination risks. This thorough examination helps ensure the safety and compliance of the manufacturing process, reducing the likelihood of product recalls and regulatory issues. In environmental monitoring, traditionally reliant on the manual counting of microbial colonies, AI introduces a level of precision through image recognition technologies. This automation standardizes colony counting, drastically reducing human error and enhancing the reliability of environmental controls.
- Chromatography, a critical technique for purifying and analyzing chemical mixtures, also benefits from AI. Traditionally dependent on manual interpretation, AI automates the integration of chromatographic peaks, significantly enhancing the accuracy and efficiency of this process. This automation is crucial for ensuring the purity and appropriate conce
- ML algorithms are now employed in the visual inspection of injectable drugs. These algorithms use advanced imaging techniques to meticulously inspect each vial or syringe for imperfections or contaminants, enhancing product quality control. This not only speeds up the inspection process but also ensures that only products that meet the highest quality standards reach patients.
- AI’s impact extends to the manufacturing of advanced therapy medicinal products (ATMPs), such as gene and cell therapies. AI technologies automate critical steps in the manufacturing process, improving the scalability and reliability of these treatments. This is especially beneficial in hospital settings, where these therapies are usually prepared and administered, making personalized medicine more accessible and practical.
These applications highlight the role of AI and ML in addressing complex challenges within drug manufacturing, driving advancements that lead to safer and more effective medications. As AI and ML continue to evolve, their integration into pharmaceuticals underscores a commitment to innovation and excellence in drug manufacturing. However, with regulators also increasingly focusing on AI, there are potential challenges for drug manufacturers, particularly in ensuring that AI-based technologies comply with applicable GMP standards. The heightened regulatory attention on AI means that AI-related tools being used for quality purposes are likely to be closely examined during inspections. Against this background, and given the developments described, it might even be expected that AI-enhanced processes will soon become the norm, and companies will be required to adapt to this new standard.
Mastering Compliance And Shaping AI Integration Into GMP Standards
Compliance with GMPs is a necessary condition for obtaining a marketing authorization.3 GMPs require manufacturers to control critical aspects of their operations through validation over the product and process life cycle. Any changes to processes affecting product quality must be documented and the impact on validation assessed.4
The importance of assuring compliance is underscored by regulators, too. For example, in the medical device context, FDA’s draft guidance entitled "Computer Software Assurance for Production and Quality System Software” notes that “software that is used as part of production or the quality system” must be validated for its intended use. Similarly, in the drug context, the EMA’s concept paper for its planned revision of GMP Annex 11 (computerized systems) states that “[t]here is an urgent need for regulatory guidance and expectations to the use of [AI] and [ML] models in critical GMP applications as industry is already implementing this technology. The primary focus should be on the relevance, adequacy and integrity of the data used to test these models with, and on the results (metrics) from such testing, rather that on the process of selecting, training and optimising the models.”
In short, manufacturers must be able to demonstrate that AI-enhanced processes are reliable and capable of ensuring GMP compliance. However, despite some emerging guidelines, as mentioned above, the existing regulatory framework has by and large not been keeping pace with the quickly advancing field of AI applications for GMP uses, especially in the pharmaceutical context. Below, we offer our thoughts on ways in which regulators’ evolving views might impact manufacturers’ use of AI and how manufacturers could deploy AI tools in a manner that is likely to withstand scrutiny.
Emerging Standards And Guidelines For The Use Of AI In Drug Manufacturing
The expansive use of AI and related concerns have led both the EU and U.S. to take steps to regulate AI.
In the EU, the AI Act5 was finally adopted on March 13, 2024, nearly three years since the proposal was published. The AI Act introduces a risk-based approach to AI systems: the higher the risk, the stricter the rules. High-risk AI systems comprise certain medical devices, which are permitted when they comply with specific requirements for adequate risk management, including human oversight and post-market monitoring.6 Other AI systems used in healthcare are not medical devices or are not considered high-risk, such as assistive technologies for elderly care, wellness apps, chatbots for triage, and public health surveillance systems.
The AI Act follows an industry-agnostic approach, meaning that it is general for all sectors and does not take into account the specificities and risks of AI applications in pharmaceuticals. Although AI finds many applications in drug manufacturing, far from all applications – if any – will be considered high-risk (or even limited or minimal risk) by the AI Act, in part because the AI Act does not apply to AI systems developed and put into service for the sole purpose of scientific research and development.
With a view to providing guidance to the life sciences sector, in particular for those AI systems that do not fall within the scope of the AI Act but which may nevertheless have an impact on the safety of patients and the integrity of data, the European Medicines Agency (EMA) has recently issued for public consultation a draft reflection paper concerning the use of AI in the drug life cycle, including manufacturing.7 This initiative is set to lead to the formulation of specific guidelines in the second half of 2024.8
With respect to manufacturing, the EMA paper provides high-level considerations, including that model development and performance assessment should follow quality risk management principles and that the ICH Q8, Q9, and Q10 principles should be considered, pending the revision of current GMPs, which is therefore taken for granted by the EMA.9 More generally, the EMA paper states that it is the responsibility of the marketing authorization holder to ensure that the algorithms, models, and data sets used are in line with good practice (GxP) standards. If the AI system may have an impact on the drug benefit-risk balance, the EMA recommends early regulatory interaction and to seek scientific advice.10 In parallel, the EMA LLFG meeting,11 explored practical applications of AI and digital technologies in drug manufacturing, as well as the regulatory challenges and solutions associated with the deployment of AI and ML in production settings. The meeting underscored the necessity of aligning AI applications with existing and evolving GMP standards.
In the U.S., the Biden administration, the FDA, and the U.S. Congress are heavily focused on AI. Among other initiatives, the Biden administration released an executive order requiring different federal agencies to adopt plans and safeguards concerning the use of AI.12 By December 2024, agencies will need to develop and implement specific safeguards to a broad use of AI applications, including health.13 Regarding pharmaceuticals, the FDA recently opened a dialog with stakeholders with the publication of two discussion papers on the use of AI in drug development and manufacturing.14 The FDA papers do not yet provide guidance, but they identify some areas on which it seeks stakeholders’ feedback. These include the need for clarity on whether and how the application of AI in drug manufacturing is subject to regulatory oversight and the need for standards for developing and validating AI models used for process control and to support release testing.15
5 Considerations For Manufacturers Using AI Applications
In the context outlined above and pending the release of specific guidelines by regulatory authorities, below are five key factors that drug manufacturers should consider when using AI in manufacturing processes to ensure robustness of their quality systems.
1. Integrity and Security of Data
Both FDA and the EMA recognize that AI is intrinsically dependent on data, which may lead to the introduction of unintended biases into models.16 Regulatory authorities also expect data to be reliable and accurate, requiring manufacturers to implement effective strategies to identify and implement measures to mitigate data integrity and security risks.17
Manufacturers should therefore ensure that the data entered into AI systems are accurate, complete, and representative of the process being monitored and controlled. This includes establishing procedures for data collection and selection, including data sources, sampling methods, and data entry processes. To ensure data integrity, manufacturers should also consider improving and adapting traditional tools, such as encryption, access controls, and audit trails, to cover potentially more large and complex data sets. Further, adequate data retention and archiving policies should be established to ensure that data is retained for the required period and retrieved for review and audit purposes. Companies need to reinforce their data security defenses by investing in advanced cybersecurity measures and establishing rigorous data governance protocols. These measures should cover the entire life cycle of data, i.e., from collection and storage to eventual disposal, and should be audited regularly to ensure adherence to stringent security standards.
2. Explainability and Transparency of AI Systems
Explainability and transparency in AI systems are crucial for maintaining trust and understanding, particularly in an industry as regulated as pharmaceuticals. The opaque nature of many AI models, especially those based on deep learning, makes it challenging for regulators to assess and verify the decision-making processes. According to the EMA, to strengthen procedural fairness, accountability, and prevention of bias, the use of transparent AI systems should be preferred.18
In anticipation of regulatory changes demanding greater transparency, companies should focus on developing interpretable AI models. Investing in explainable AI (XAI) technologies and keeping detailed documentation of AI decision-making processes will be critical. Such efforts will not only aid in regulatory inspections but also enhance the overall reliability and acceptance of AI applications in drug manufacturing.
3. Validation of Protocols and Testing Acceptance
Manufacturers must control critical aspects of their operations through validation over the product and process life cycle.19 AI/ML specific features such as continuous learning pose significant challenges as AI systems continuously evolve based on new data. This ability to learn can affect the consistency of AI performance, complicating compliance with the traditionally static expectations of GMP standards. In response, regulators might update frameworks like the EMA’s Annex 11 to encompass continuous monitoring and re-validation protocols for AI systems.
Companies should implement robust change control systems to manage updates to AI algorithms and monitor their outputs continuously. When using AI systems, manufacturers should consider developing new validation protocols or improving existing ones that define the objectives, scope, and acceptance criteria for validating AI systems. This may include performance testing, comparison against reference methods, and evaluation of algorithm robustness. To ensure that AI-based processes work as intended and meet regulatory requirements, adequate procedures for testing their integral performance should be established or enhanced, for example, by testing individual components of the system against applicable standards.20 By maintaining detailed records of all algorithmic changes and performance metrics, firms can ensure ongoing compliance with regulatory standards that are adapting to this dynamic landscape.
4. Management of AI-Related Risks
The use of AI brings new and unknown types of risks which may not be foreseen in existing frameworks. For example, if algorithms are not tested for potential errors, there is a risk of unfair or unreliable results, such as false-positives/false-negatives, which could lead to data integrity issues if proper controls are not in place to manage data input and oversight.21 These risks need to be assessed and mitigated appropriately.
Manufacturers should therefore put in place a risk management plan. A thorough risk assessment to identify potential risks stemming from the use of AI in quality should be carried out, following which adequate risk mitigation measures should be implemented to reduce or eliminate identified risks. This may require adopting specific controls, enhancing cybersecurity measures, and establishing contingency plans for system failures. And since AI is a constantly evolving area, continuous monitoring and review are necessary to ensure that mitigation measures remain effective and any new risks are addressed in a timely manner.
5. In-House Resources Training and Competence
Adequate resources should be devoted to personnel training. The use of AI still largely relies on human oversight, and some human oversight is required for the use of high-risk AI systems, as mandated in the AI Act.22 The GMP standards state that the correct manufacture of drugs relies upon people.23 For this reason, sufficient qualified personnel must be present to perform tasks that are the responsibility of the manufacturer.
Manufacturers should therefore consider developing training programs to ensure that personnel using or overseeing AI-based applications are adequately trained and competent to carry out their tasks effectively. Besides the basic notions on the quality management system and GMPs, existing and newly recruited personnel should receive adequate training for the tasks assigned to them, including on AI fundamentals and any other aspects concerning the specific AI system deployed.
Conclusion
By proactively addressing the described challenges, pharmaceutical companies may not only comply with evolving GMP standards but also harness the full potential of AI to innovate and improve manufacturing processes. By focusing on the use of best practices, innovative technologies, and effective management strategies, operational excellence will not only optimize production processes and compliance but will eventually have a significant impact on patient health and safety.
References
- Commissioner Robert M. Califf, Harnessing the Potential of Artificial Intelligence, March 15, 2024, available here.
- See meeting report relating to the EMA 2nd Listen and Learn Focus Group (LLFG) meeting of the Quality Innovation group (QIG) held on Oct. 12-13, 2023, available here.
- In the EU, see: Directive 2001/83/EC (Title IV), Commission Directive (EU) 2017/1572, and Commission Delegated Regulation (EU) 2017/1569. See also European Commission EudraLex Volume 4. In the U.S. see: 21 CFR Parts 210, 211, 820.
- See Annex 15, European Commission EudraLex Volume 4.
- Proposal for a Regulation laying down harmonized rules on Artificial Intelligence (Artificial Intelligence Act) and amending certain Union Legislative Acts (COM(2021)0206 – C9-0146/2021 – 2021/0106(COD)).
- See Study on Artificial intelligence in healthcare, Scientific Foresight Unit (STOA) of the European Parliamentary Research Service, June 2022, available here.
- EMA draft Reflection paper on the use of AI in the medicinal product lifecycle, available here
- See EMA-HMA workplan to guide use of AI in medicines regulation, available here.
- See Section 2.2.6, EMA reflection paper.
- See Section 2.5, EMA reflection paper.
- See footnote No. 2 above.
- Executive Order on the Safe, Secure, and Trustworthy Development and Use of Artificial Intelligence, White House, Oct. 30, 2023, available here.
- Vice President Harris Announces OMB Policy to Advance Governance, Innovation, and Risk Management in Federal Agencies’ Use of Artificial Intelligence, White House, Mar. 28, 2024, available here.
- FDA Discussion Paper on Using Artificial Intelligence and Machine Learning in the Development of Drug and Biological Products (May 2023), and FDA Discussion Paper on Artificial Intelligence in Drug Manufacturing (February 2023). The discussion papers were open to consultation and comments from stakeholders.
- See Sections 3 and 4, FDA Discussion Paper on Artificial Intelligence in Drug Manufacturing.
- See Section 2.4.1, EMA Reflection Paper, and FDA Discussion paper on Artificial Intelligence in Drug Manufacturing.
- See FDA, Guidance for Industry, Data Integrity and Compliance With Drug CGMP (December 2018), available here.
- See Section 2.4.5, EMA Reflection Paper.
- See Annex 15, European Commission EudraLex Volume 4. See also FDA, Guidance for Industry, Process Validation: General Principles and Practices (January 2011), available here.
- See Section 2.4.2, EMA Reflection Paper.
- See World Health Organization, Benefits and risks of using artificial intelligence for pharmaceutical development and delivery, available here.
- See Article 14, AI Act.
- See Chapter 2, European Commission EudraLex Volume 4.
About The Lead Author:
Josefine Sommer is a partner in Sidley Austin’s Global Life Sciences practice based in Brussels. She assists clients in regulatory, compliance, and enforcement matters. She counsels medical device, pharmaceutical, and biotech companies on EU regulatory compliance, including in clinical trials, authorizations, and regulatory authority interactions. She additionally advises clients on EU environmental law, including chemicals legislation impacting medical devices, pharma, and biotech, as well as consumer products. Sommer handles GMP and quality management system (QMS) matters, and also represents companies in regulatory enforcement actions.