A Dose Of Accuracy In Precision Medicines: Limitations Of Precision Medicines
By Dr. Sanjay Srivastava, Managing Director, Cell & Gene therapy lead and Eric Clark, Ph.D., consultant at Accenture
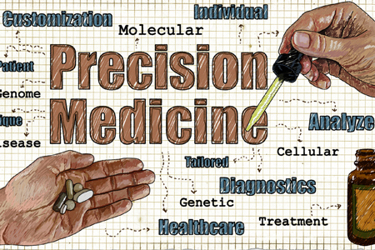
According to the U.S. National Research Council (NRC), precision medicine is defined as “the tailoring of medical treatment to the individual characteristics of each patient”.1 In line with this goal, the medical field started tailoring treatments to smaller cohorts by profiling genetic or molecular markers. The result is less variability between patients, thereby increasing precision of the therapy. Autologous CGTs have proven to be one of the most promising precision medicines in the cancer field, supported by a recent published article showing long term remission of patients treated 10 years ago2.
Even with these CGT successes, precision medicines, including Cell and Gene Therapies (CGTs) aren’t accurate enough to consistently deliver desired outcomes for all patients within the defined subgroups. Medical staff are limited in being able to predict and differentiate between responders and non-responders. To improve outcomes for all patients, it is necessary to design unique treatments for everyone. Precision medicines, when individually personalized would activate accurate medicine by driving consistent and predictable desired outcomes and essentially transforming the way we can treat diseases such as cancer.
Requirements to personalize at the patient level include 1) an in-depth understanding of a disease and its risk factors / component causes, something the research industry is continuously striving for; 2) improve personalization through analysis of the patient’s systems biology, demography, medical histories, responsiveness to medications and other characteristics; 3) an ability to target the disease cause / risk factors using precision tools such as CRISPR and other innovative methodologies.
Recent capabilities for points 2 and 3 help biopharma companies to start developing an accurate medicine strategy (Figure 1). This strategy not only considers individual differences in people’s genes but also individual patient’s unique biology and requirements throughout the treatment process.
The definitions from Figure 1 are emphasized when we look at these factors in the context of Jessie Gelsinger’s death in 1999. The tool used in the trial was an adenovirus, which can trigger a high immune response3. The immune response may differ between patients suggesting low treatment precision. In the investigation of Jessie’s death, findings suggested that Jessie had poor liver function and should not have received the therapy3. This was a lack of personalization of the therapy to Jessie’s specific biology.
Figure 1: Definitions of One Drug Fits All, Precision Medicine, and Accurate Medicine. Bullet points demonstrate key capabilities that facilitate Precision Medicine and Accurate Medicine strategies. Precision and personalization are enablers of more accurate medicine. Copyright © 2022 Accenture.
Another important component of personalization are the operational considerations surrounding the patient, including informed consent. Jessie was not informed of side effects experienced by previous patients or the death of two monkeys during pre-clinical studies3. Finally, the therapy was inaccurate because the disease was not treated. Since this tragic incident, the field has been dedicated to creating better precision tools and personalized strategy, leading to more accurate medicine. Regulatory oversight and patient advocacy strategies have recently evolved to improve patient safety while judiciously allowing these personalized, transformative therapies to go to market.
Using biomarkers and analytics to understand systems biology and personalize patient therapies
The biopharma industry has realized that a “One Drug Fits All” approach leads to costly failures in treatment development. One report quantified that an anti-cancer drug was ineffective for 75% of patients4. Phase I and II clinical trials start with smaller populations to ensure safety and then scale to larger and larger groups for Phase III, IV, and commercial use. Expanding the treatment to larger and larger patient groups introduces more variability for treatment response, which can lead to dangerous side effects. As the treatment group grows, tailoring treatments to specific cohorts facilitates precision medicine. Understanding each patient’s systems biology facilitates precise disease treatment while mitigating side effects and activates accurate medicine.
Understanding a patient’s systems biology requires a large data volume. Increasingly, continuous real time data is collected from patients and their environment. Examples of the data collected throughout the patient journey can include genomic and other -omics, cytokine levels, histology analyses, serum levels, hematology tests, medical histories from for the patient and their family, patient demographics and registry information, apheresis and infusion dates, pre infusion vitals, informed consent, clinical notes, apheresis product processing, cell sample processing, certificate of analysis, viral vector copy number and quality (if using a viral delivery strategy), cell culture and sample characteristics, adverse events, and more. Most of this data serves as patient biomarkers that can be tracked throughout their treatment journey.
One powerful example of a multi-omics approach to better understanding a patient’s systems biology is using single cell assays. In this approach a single cell’s genotype and disease phenotype information can be measured for multiple cell types across different patient samples, improving personalization and patient stratification5. This is particularly important for understanding a specific cell type of interest to be targeted in an in-vivo gene therapy. Understanding a cell’s biology before and after gene alteration can help uncover on and off target effects at a cellular level to estimate the efficacy and safety of a therapy better than ever before. This powerful technology is making its way into clinical trials which could greatly improve our understanding of a patient’s disease and how to treat it6.
Continuously capturing patient data through a living lab facilitates personalized medicine
Understanding a patient’s systems biology and how it relates to the disease is crucial for accurate medicine. However, the data should be appropriately captured and analyzed cumulatively with other data types, including operations data throughout the value chain, to create a digital thread for consistent data intake. In addition to the drug discovery and development considerations mentioned above, data collection from supply chain, manufacturing, and commercial operations is crucial. Enterprise data management from the wide variety of sources is possible using a ‘Living Digital Lab’ (Figure 2). Automating the capture of patient operational data leads to better consistency and continuous data gathering, facilitating an evidence-based improvement in treatment strategy over time.
After creating a living lab that captures data from disparate data sources, data, integration, retrieval, and analysis is required to understand how the patient’s data is related to disease and to create a personalized treatment strategy. By creating a living digital lab with data securely housed in a centralized platform and embedded AI and analytics capabilities, patient insights are generated in real time and can be acted on faster and throughout the treatment journey.
Figure 2: Insights and capabilities from the ‘Living Digital Lab’ create new opportunities across the enterprise and allow continuous learning to keep improving patient treatment strategies. Copyright © 2022 Accenture.
In our model, wholistic patient data is transformed and cleaned to ensure standardization of data formats in the temporary storage location such as the Data Trust, Clinical Data Warehouse, ERP, MES, or NIMS systems. The data is de-identified and stored in a data lake. AI/ML strategies allow analysis of complex data types to create patient insights (Fig 3).
Large amounts of de-identified data are needed to generate powerful patient insights, necessitating access to large amounts of patient data. Federated learning is a strategy where an algorithm can be trained on multiple data sources to get collaborative insights while ensuring patient privacy and preserving intellectual property7. Using federated strategies, internal and external data can be analyzed to sufficiently power patient insights. More patient data enables prescriptive analytics and simulation of treatment outcomes to select the most successful, optimized CGT strategy for individual patients. Access to more patient data helps creates robust synthetic control arms (SCAs) which could essentially create a virtual control cohort for comparing patient treatment outcomes8. SCAs reduce the demand for patient recruitment to save time and resources while de-risking clinical trials. Ultimately, a more data driven, personalized approach facilitated by federated learning, prescriptive analytics, and SCA capabilities allows a better chance for high efficacy therapies while mitigating potential harmful side effects, which promotes more accurate medicine.
Figure 3: Data ingestion and aggregation strategy from biomarker and operational data types to improve patient insights. Copyright © 2022 Accenture.
A data-driven therapy created for a specific patient, based on their internal (systems) and external risk factors, combined with operational considerations throughout the patient journey, provides a thorough understanding of a patient’s disease and how/when to deliver the treatment.
Precision: Targeting the patient’s risk factors with gene-editing technologies
Once a treatment strategy is determined and personalized for the patient based on the analysis from the living lab, the appropriate gene editing technology is required to precisely target the disease risk factors and attempt to cure the disease. Viral vectors (retrovirus, lentivirus, AAV, etc.) and genome editing nucleases (CRISPR, TALENS, ZFNs) can target DNA by a distinct complementary sequence within the gene of interest to decrease disease risk (measured by disease biomarkers) for better target efficacy and to limit off-target effects. These genome editing nucleases are highly precise and facilitate better specificity for treatment strategies.
For example, a recent study suggested that over 25% of known pathogenic point mutations listed in ClinVar, a public database developed by the U.S. National Institutes of Health (NIH), could theoretically be corrected using base editing CRISPR technology9. This new CRISPR technology that, rather than cutting both DNA strands, only cuts one strand which allows more precise editing. Prime editing is an upgrade to base editing technology and could allow for treatment of 90% of the pathogenic mutations listed in ClinVar10.
Precision tools help facilitate accurate medicine for different types of CGTs. For ex vivo allogenic CGTs, a donor cell can be edited using CRISPR technology to reduce the immune response when given to a patient, creating potential for universal donor cells used for allogenic therapies. This approach both increases precision by limiting the chance of harmful side effects and mitigating high COGS associated with autologous therapies. For in-vivo therapies, CRISPR/Cas9 can be delivered in lipid nanoparticles to a specific cell type within the patient by binding to cell type specific receptors11. Once inside a cell, the nanoparticle contents are released. DNA targeting therapies like CRISPR must then enter the nucleus to bind to the recognition sequence and make the required gene edit suggested by the ‘Living Lab’ analysis. Targeted delivery and gene editing technologies allow a precision not previously available to the CGT field.
Accurate Medicines: Treatments and preventions developed uniquely for everyone
Accurate medicine is enabled when precision medicines are delivered with a personalized strategy. For example, a universal allogeneic cell source could be edited with specific patient considerations in mind to provide the highest possible accuracy. UPenn is leveraging deep learning into their studies to fine tune the way patients are treated with CAR T cells12. For in-vivo viral vector gene therapies, artificial intelligence (AI) can be used to select the correct viral vector for the individual patient. This fine tuning to individual patient’s needs is the essential push to advance beyond precision medicines at a cohort level to a truly accurate way of treating ALL patients.
One of the best examples of an accurate medicine strategy is using a precision gene editing strategy to target disease early based on ‘Living Lab’ patient insights. This approach requires an in-depth understanding of a disease and how risk factors cumulatively contribute to cause the disease. Real-time data is captured by the ‘Living Digital Lab’ and securely stored. The data is analyzed and referenced to the federated database system (FDBS) to identify internal (e.g., genomic, and multi-omic profile) and external (e.g., demographics, diet) risk factors that contribute to the cause of the disease. A treatment strategy is developed based on risk factors and data from the entire value chain including R&D, manufacturing, supply chain, and commercial data, determining when and how the treatment is administered. During treatment execution, CRISPR or other precision tools are delivered ex vivo to the patient cells or in vivo to the patient to target the correct cell type and make the specific gene edit that prevents the disease before symptom onset. This accurate medicine strategy contains the capabilities to treat individual patients earlier, facilitating more common, multi-genic diseases to be targeted in a preventative approach.
In conclusion, accurate medicine is the ultimate goal that CGT innovators should strive for. It requires an interdisciplinary approach driven by biology, operations, and analytics. By implementing the correct precision and personalized therapeutic capabilities (internally or externally through partnerships), we can improve clinical outcomes for all patients.
Biographies:
Eric Clark, Ph.D., is a consultant in Accenture’s Life Sciences Strategy & Consulting practice. With experience in cell biology and genetics research and consulting experience in the R&D domain, Eric has accumulated 10 years of experience in the life science industry. Eric works with biopharma clients to improve research and clinical development strategy, processes, and technology. His focus is cell and gene therapies and increasing treatment accuracy to facilitate improved patient outcomes.
Dr. Sanjay Srivastava, Managing Director, Cell & Gene therapy lead at Accenture. Sanjay has a broad and deep expertise in the underpinning science and business challenges in developing and launching both in-vivo and ex-vivo cell & gene therapies. He directs his C> team at Accenture, who has global expertise in R&D, supply chain, manufacturing, and commercial operations.
Acknowledgements:
Thank you to Phillip An and Marcus Carpentier for their revisions to this article.
Disclaimer:
This content is provided for general information purposes and is not intended to be used in place of consultation with our professional advisors. This document refers to marks owned by third parties. All such third-party marks are the property of their respective owners. No sponsorship, endorsement, or approval of this content by the owners of such marks is intended, expressed, or implied.
Resources:
- https://pubmed.ncbi.nlm.nih.gov/22536618/
- https://www.nature.com/articles/s41586-021-04390-6
- https://www.sciencehistory.org/distillations/the-death-of-jesse-gelsinger-20-years-later
- https://www.ncbi.nlm.nih.gov/pmc/articles/PMC4766785/
- https://www.cellandgene.com/doc/how-single-cell-multi-omics-will-accelerate-cell-and-gene-therapy-development-0001
- https://www.nature.com/articles/d41591-019-00017-6
- https://www.accenture.com/us-en/blogs/technology-innovation/giordano-dey-driving-collaboration-with-privacy-preserving-computation
- https://www.accenture.com/us-en/insights/life-sciences/faster-cheaper-clinical-trials
- https://www.ncbi.nlm.nih.gov/pmc/articles/PMC7503568/
- https://www.genomeweb.com/gene-silencinggene-editing/broad-institutes-new-prime-editing-tech-corrects-nearly-90-percent-human#.YmXFQ9rMK5c
- https://www.nature.com/articles/s41573-020-0075-7
- https://www.pennmedicine.org/news/news-releases/2022/february/study-of-penn-patients-with-decade-long-leukemia-remissions-after-car-t-cell-therapy